This One-Way ANOVA Calculator helps you compare means between three or more groups to determine if there are statistically significant differences. For example, you could compare test scores between multiple teaching methods, or analyze plant growth under different conditions. The calculator performs comprehensive statistical analysis including assumption checks (normality, homogeneity of variance), effect size calculations, and generates publication-ready APA format reports. To learn about the data format required and test this calculator, click here to populate the sample data.
Calculator
1. Load Your Data
2. Select Columns & Options
Related Calculators
Learn More
One-way ANOVA
Definition
One-way ANOVA (Analysis of Variance) tests whether there are significant differences between the means of three or more independent groups. It extends the t-test to multiple groups while controlling the Type I error rate.
Why Do We Need ANOVA?
When comparing multiple groups, you might be tempted to perform multiple t-tests between all possible pairs of groups. However, this approach leads to a serious problem: an increased risk of Type I errors (false positives) .
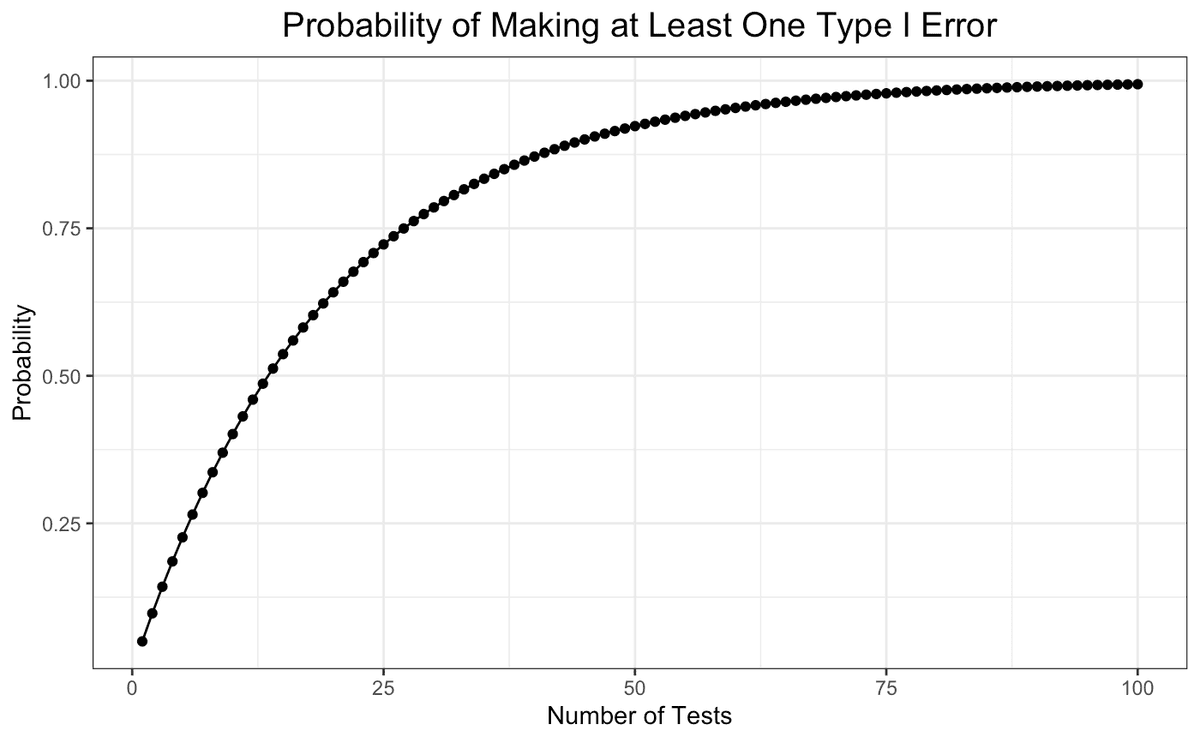
- With 3 groups (3 pairwise tests): 14.3% chance
- With 4 groups (6 pairwise tests): 26.5% chance
- With 5 groups (10 pairwise tests): 40.1% chance
Interactive ANOVA Explorer
Configure Groups
Group 1
Group 2
Understanding ANOVA Scenarios
Large mean difference (8 vs 11) + Small spread (SD=0.5) = Strong evidence of group differences
When group means are far apart and individual measurements have minimal variation, differences become clearly distinguishable.
Small mean difference (8 vs 9) + Small spread (SD=0.5) = Moderate evidence of group differences
When group means are relatively close but individual measurements show little variation, meaningful differences may still be detectable.
Large mean difference (8 vs 11) + Large spread (SD=2) = Weak evidence of group differences
When individual measurements vary widely within groups, even substantial differences between group means can be difficult to detect reliably.
Note: This is a visual demonstration only.
The "evidence strength" indications are simplified visual examples to illustrate ANOVA concepts. No actual statistical test is being performed here. In practice, an ANOVA test would calculate specific statistics (F-ratio, p-value) to formally evaluate the evidence for group differences.
Current scenario: Strong evidence of group differences
Formula
Key Components:
Between-groups sum of squares, where:
- = mean of group
- = grand mean
- = sample size of group
Within-groups sum of squares, where:
- = th observation in group
- = mean of group
- = sample size of group
Final Test Statistic:
Where:
- = within-groups sum of squares
- = number of groups
- = total sample size
Key Assumptions
Practical Example
Step 1: State the Data
Group A | Group B | Group C |
---|---|---|
8 | 6 | 9 |
9 | 5 | 10 |
7 | 8 | 10 |
10 | 7 | 8 |
Step 2: State Hypotheses
- (all means equal)
- at least one mean is different
Step 3: Calculate Summary Statistics
- Group A:
- Group B:
- Group C:
- Grand mean:
Step 4: Calculate Sums of Squares
Step 5: Calculate Mean Squares
Step 6: Calculate F-statistic
Step 7: Draw Conclusion
The critical value at is .
The calculated F-statistic () is greater than the critical value (), and the p-value () is less than our significance level (). We reject the null hypothesis in favor of the alternative. There is statistically significant evidence to conclude that not all group means are equal. Specifically, at least one group mean differs significantly from the others.
Effect Size
Eta-squared () measures the proportion of variance explained:
Guidelines:
- Small effect:
- Medium effect:
- Large effect:
For the example above, the effect size is:which indicates a large effect.
Code Examples
library(tidyverse)
group <- factor(c(rep("A", 4), rep("B", 4), rep("C", 4)))
values <- c(8, 9, 7, 10, 6, 5, 8, 7, 9, 10, 10, 8)
data <- tibble(group, values)
anova_result <- aov(values ~ group, data = data)
summary(anova_result)
import numpy as np
from scipy import stats
group_A = [8, 9, 7, 10]
group_B = [6, 5, 8, 7]
group_C = [9, 10, 10, 8]
# Perform one-way ANOVA
f_stat, p_value = stats.f_oneway(group_A, group_B, group_C)
# Print results
print(f'F-statistic: {f_stat:.4f}')
print(f'p-value: {p_value:.4f}')
Alternative Tests
Consider these alternatives when assumptions are violated:
- Kruskal-Wallis Test: Non-parametric alternative when normality is violated
- Welch's ANOVA: When variances are unequal
- Brown-Forsythe Test: Robust to violations of normality